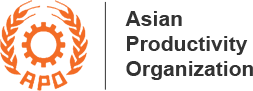
Select Page
In those conventional irrigation projects, multiple pumps are used to carry water from primary to secondary and tertiary channels. However, convenience losses occur in natural canals and energy losses occur during long-term pump operations. On the other hand, the soil moisture and water requirements change significantly based on the soil type, existing moisture content, types of organic matter present in the soil, and slope of the land for water retention. In addition, field capacity and plant wilting points make water requirements variable.
Applications of deep learning and Internet of Things (IoT)-based technologies offer solutions to farmers and large-scale irrigation project managers who must with differing soil moisture levels, types of organic matter, and slope in irrigated areas. Deep learning-based IoT-controlled pump operations have the potential to enable optimal, site-specific operations. Single pumps in a system can be activated only when needed in areas where water demand is the highest. High-throughput phenotyping using drones and wireless (WiFi) distributed soil moisture sensors can provide information on which locations require pump operation. Thermal imagery is a promising method for determining moisture content using drones to collect images from the field (Figure 1). Thermal image processing with an orthomosaic datasets can be utilized to develop water-stress maps, which help in recognizing areas where crops lack sufficient water.
In IoT-based irrigation management, low-cost WiFi modules, relays, and microcontrollers operate water pumps (Figure 2). Pumps are connected via relays to microcontrollers, and the microcontrollers are in turn connected to WiFi modules. The WiFi modules transmit signals from the field to a narrowband (NB)-IoT controller, which can then be accessed through a smartphone app. NB-IoT systems ensure WiFi signal generation using low-power wide-area networks (LPWANs).
Figure 2. Connection diagram for a water pump operation system using low-cost relays, a microcontroller, and WiFi module. (Source: Author)
In a deep learning system, convolution neural network (CNN) ensures accuracy based on the training datasets. The historical datasets and in-situ soil moisture information of large irrigation command areas can be classified based on soil type, organic matter content, and slope. The first classification includes the water retention capacities of major soil types: sandy; silty; and clay loams. The second is related to the high, medium, or low organic matter presence in the soil. The third classification is the slope of the land, which can be determined from raster-generated maps using GIS spatial analysis. In addition, the water requirements of crops can be calculated using remote sensing systems that detect water stress indices based on shortwave and near-infrared data from the Landsat 8 Operational Land Imagery.
Water stress classifiers can also be included in the training datasets, categorized as high, medium, and low stress taking into account phenology indices, plant water requirements, evapotranspiration rates, and deep percolation of water. All three major classifiers using deep learning procedures require a large dataset for training to develop an NB-IoT and deep learning-based water management system to minimize water losses during irrigation.
There are multiple advantages of such advanced irrigation management systems in both developed and developing countries. Pump operational times can be reduced, which also saves on energy consumption. According to site-specific variations and water requirements, selected pumps can be operated only in required locations. Systems using a distributed NB-IoT are promising methods for the development of smart irrigation systems. Distributed WiFi modules and in-situ ground reference soil moisture sensors allow remote, real-time confirmation of soil moisture levels in the field. Therefore, Asian countries adopting smart irrigation systems can expect to save water while increasing rice and other crop production.
Dr. Tofael Ahamed is the expert for the APO e-learning course on Smart Farm Mechanization. Course description is available here.
Tofael Ahamed is an Associate Professor, Faculty of Life and Environmental Sciences, University of Tsukuba, which is one of the leading universities in Japan. Dr. Ahamed has also teaching and research experiences as Lecturer, Assistant, and Associate Professor in the Department of Farm Power and Machinery, Bangladesh Agricultural University. He received PhD from the University of Tsukuba and conducted postdoctoral research at the University of Illinois at Urbana-Champaign, USA.
Dr. Ahamed performs research in the field of precision agriculture technology, agricultural robotics and decision support systems using GIS and satellite remote sensing. In the sensing system development, Dr. Ahamed focuses on enabling smart application of IoT in agriculture, where crop production varies spatially and temporally within the field boundaries depending on the soil and environmental conditions.